No matter who you ask, everyone agrees that draft preparation is one of the most important parts of a fantasy baseball season. Yes, a main part of preparation is knowing which players you are targeting and when to target them. But what happens when you miss out on your targets? What happens when the few specific players you want to build around are taken? By using a combination of projection systems and category benchmarks, you can fill in gaps during your draft based on what you expect you’ll need to come out ahead in each category.
Before we go any further, despite this article being in a series of articles titled Fantasy 101, this article is more of a 300-level elective as opposed to a core curriculum course. While preparing for drafts at the beginning of your fantasy career, knowing how to leverage projection systems and benchmarks will put you ahead of other starting players, but so will a careful study of ADP and fantasy baseball experts. Many of the skills covered in this article will help prepare you for deeper leagues or auctions with a little finessing. Some modicum of experience working within Excel or Google Sheets is a prerequisite for taking full advantage of what is covered in this article. An example spreadsheet has been provided to allow readers to follow along.
Projection Systems
Despite confidence to the contrary, we are all continuously learning what leads to success on the baseball field. While Sabermetrics has been growing for decades and we certainly know more than we used to, this game is still played by people who could over or under-perform their projections for a myriad of reasons that won’t be captured by a calculation.
That doesn’t mean that we will stop trying! The various projection systems out there (either in antiquity, in current use, or on the drawing board) are all attempts at inferential statistics – inferring the future from the past. Each projection system uses some form of historical baseball data to try and determine how a player may perform the next season.
For each system, you must accept there are assumptions made and outside forces at play. The parties putting together each system are making educated guesses about position eligibility and playing time based on MLB team roster construction and the perceived value (and injury risk) of each player. None will be exactly right (in fact, most hover somewhere near an R2 of 0.6), but they do help provide a fantasy manager some forward-looking assumptions to build a roster around. For those unfamiliar with R2, it is a -1 to 1 measure which describes how well the linear regression fits the data, where a stronger correlation is at the boundaries and it gets progressively weaker as you approach 0.
What Systems are Out There
Projection System | Location | Description |
---|---|---|
Marcel | Baseball-Reference | Developed by Tom Tango, this is the base system most players start with. Using the last 3 years of MLB data, weighted by proximity to the current year, Marcel calculates a regression towards the mean for each category. Age adjustments are applied. More details on Tango’s system are available here: http://tangotiger.net/marcel/ |
ZiPS | Fangraphs | Developed by Dan Szymborski, “ZiPS uses growth and decline curves based on player type to find trends. It then factors those trends into the past performance of those players to come up with projections.” Details about the ZiPS system are available here: http://m.mlb.com/glossary/projection-systems/szymborski-projection-system |
Steamer | Fangraphs | Developed by Jared Cross, “Steamer uses past performance and aging trends to develop a future projection for players. It also uses pitch-tracking data to help forecast pitchers.” For more information about Steamer, stop here: http://m.mlb.com/glossary/projection-systems/steamer |
The BAT | Fangraphs | Initially developed as a DFS projection system, Derek Carty from RotoGrinders implemented a seasonal projection from his original model. Per Carty, “THE BAT incorporates all the necessary basics, like park factors and platoon splits, plus many lesser-considered factors like air density and umpires to give you an edge on the competition.” Visit RotoGrinders for more details about The BAT: https://rotogrinders.com/marketplace/derek-carty-s-the-bat-projection-system-300 |
Depth Charts | Fangraphs | Developed by Fangraphs, the Depth Charts system is a blend of Steamer and ZiPS scaled by playing time expectations. The original article detailing the Depth Charts system is available here: https://library.fangraphs.com/depth-charts/ |
Pod | Projecting X – Additional Cost | The Pod projections are one of the several tools offered by Mike Podhorzer on the Projecting X website. If you are interested in the methods used to develop the Pod projections system, there is a Projecting X 2.0 ebook available for purchase. |
PECOTA | Baseball Prospectus – Additional Cost | The Player Empirical Comparison and Optimization Test Algorithm is a proprietary system developed by Nate Silver while at Baseball Prospectus. There is a lot of secret sauce to the process, but in general, the system finds players with similar historical trends and projects a seasonal figure based on what those other players in history were able to do. |
ATC | Fangraphs | Developed by Ariel Cohen, the ATC projection system is a machine that consolidates and weights other projection systems based on their historical accuracy. For instance, System A may be given a 20% weight for batter home runs, but only 5% for pitcher strikeouts. Ariel Cohen provides a primer on his system here: https://fantasy.fangraphs.com/the-atc-projection-system/ |
Comparing the Systems
On this topic, I stand upon the shoulders of others. Mr. Cheatsheet and Fantasy Pros rank the effectiveness of each baseball projection system at the conclusion of each season. It’s important to know that the rankings for each system will fluctuate year-to-year, but there are general trends (e.g one system is historically better at pitching) you can discern.
Mr. Cheatsheet’s 2019 analysis can be found here: http://mrcheatsheet.com/2019/03/09/projection-analysis-2019/
Ariel Cohen’s take on 2018 projection system rankings is available here: https://fantasy.fangraphs.com/2018-projection-systems-comparison-a-game-theory-approach/
FantasyPros 2018 projection system rankings are available now. The rankings for 2019 should come out in March if history holds. The 2018 article is here: https://www.fantasypros.com/2019/03/most-accurate-fantasy-baseball-projections-2018/
Which Projection System Is Best (For My Needs)
Considering the last section demonstrates that the accuracy of each system varies year-to-year and even position-by-position, I often find that ATC or Depth Charts are my two go-to projections (also helps that they are both free). ATC is a bit of a black box on how it chooses which assumptions to keep from each system, but the fact that it blends systems still helps rough out the edges from any one source. Depth Charts only uses two systems (Steamer and ZiPS), but you can plainly see the assumptions that it makes for the blend (e.g. playing time), so it helps assuage the fear of not knowing what is going on.
Benchmarks to Hit
A general rule of thumb for rotisserie leagues is that you want to strive for third-place in every category. In a 12-team roto league, that would equate to 100 points on the season – 83% of the maximum score possible in the league. Since you’ll be hypothetically fielding a balanced team across all of the scoring categories, you are now banking on one other person to have a team 1-17% better than you cumulatively, no small task. That same rule is applicable to head-to-head, but you need to take into account the streakiness of a player when making your choices. In either case, you need to know how many Runs or what ERA would be good for third-place in your league!
As stated in the introduction for this article, this is the section that requires some use of advanced math and spreadsheets (which really does a great job obfuscating the math). If you want to brush up on linear regression (which we use the simplest form of), there are some good resources online, such as http://onlinestatbook.com/2/regression/intro.html. Similarly, the R2 (Pearson’s correlation) is explained here.
Calculating Your Targets
To start this section, you must remember one thing – more data is better data. Specifically, more data that matches each specific league type will help inform your decisions for the upcoming draft. Most of the fantasy platforms will release an article during draft season with targets to hit to win your league. Their information is based on all of the histories of their application they have stored in their proprietary system, which means that it’s valuable, but also a black box that can’t be adjusted to fit your league needs.
Let’s say you are in standard Yahoo Head-to-Head Points league with 12 teams. Your goal should be to collect the league results for that league type from as many leagues and seasons as possible. What this information gives you is the ability to calculate your targets for each category with better control for outliers that may end up skewing your figures. In the end, you’ll have a data set that matches your league experience (likely with the same league mates) that includes their tendencies.
For the purpose of this article, Google Sheets was used providing a link to a working example of the process.
Step 1: Separate the Data by Category and Calculate the Ranks
As a matter of preference, I have opted to create a separate tab in my Google Sheet for each category. For each league you have information from, copy the appropriate category’s team values into the totals column. In the ranks column, use the RANK function for each value (within each league) as compared to the values for the league as a whole.
=RANK($A2, $A$2:$A$13, 1)
The function above will provide the RANK for the table below, as compared to all of the values between 961 and 825. The 1 in the third parameter indicates that the rank should be ascending (a higher number for the higher total value). For each league and season in your data, you’ll need to calculate the rank for each league and season separately! If there was another league season in that same spreadsheet, the equation would be:
=RANK($A14, $A$14:$A$25, 1)
This is the most time-consuming step of the process since you can’t simply drag the equation (as written) down the whole list of totals.
Totals | Rank |
---|---|
961 | 12 |
878 | 6 |
778 | 3 |
960 | 11 |
757 | 1 |
880 | 7 |
887 | 8 |
896 | 10 |
838 | 5 |
894 | 9 |
760 | 2 |
825 | 4 |
Step 2: Visualize the Data (Check Your Work)
A fairly easy primer for doing a simple regression in Google Sheets can be found here: https://scholarlyoa.com/regression-using-google-sheets/
For this step (and step 3), you’ll be venturing into the statistical form known as linear regressions. A linear regression models the relationship between a dependent variable (rank in this case) and an independent value (the team totals).
Following the steps in the primer that is linked above, I can see a relationship between rank (1-12 based on a 12 team league) and the WHIP for each team at those ranks. In this example, the R2 (a -1 to 1 measure that describes how well the linear regression fits the data) is 0.93, indicating a very strong relationship between team totals for WHIP (across 6 teams in the 2019 season) and their rank.
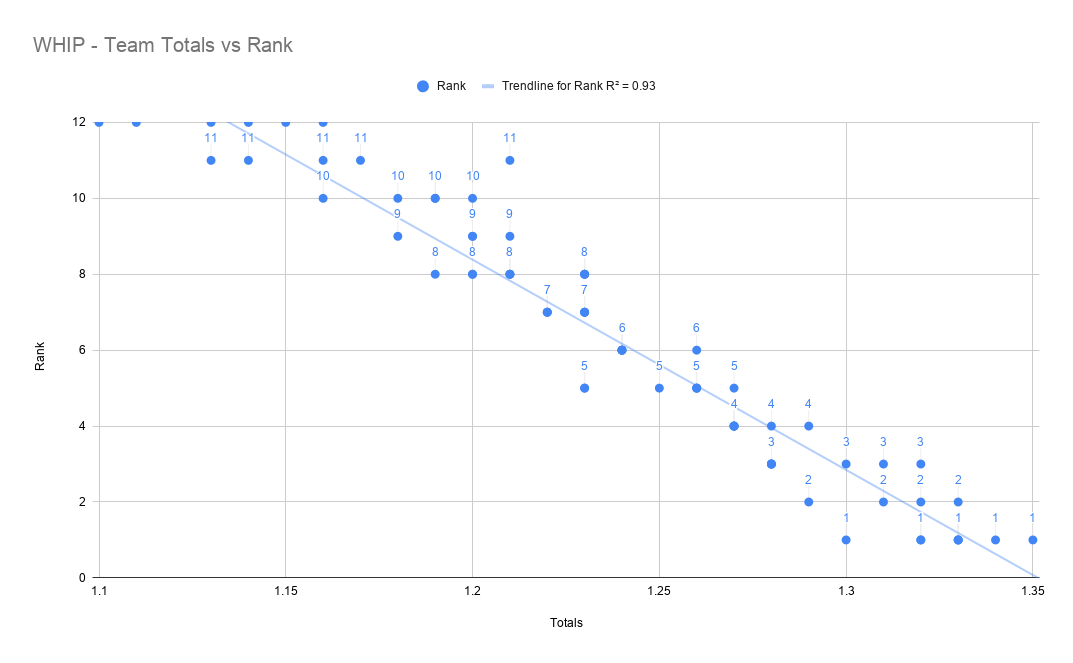
WHIP vs. Ranks
Step 3: Calculate the Per Rank and Third Place Values
Instead of needing to generate a graph (like the one in Step 2) and guess what the third-place value (equivalent to the 10 rank) and the difference necessary to go up a rank for each category, Google Sheets provides two easy formulas to generate those numbers.
The Slope – Per Rank Value
The slope of the line from the linear regression is the equivalent needed to move from one rank to the next rank for each category. The slope number assumes that you also know the intercept, which would be the equivalent of what someone with a 0 rank would have tallied per category.
=SLOPE(R!A:A, R!B:B)
The calculation above assumes that in the sheet named R in the workbook, the independent variable (team totals) are in column A and the dependent variable (ranks) are in column B.
=INTERCEPT(R!A:A, R!B:B)
The calculation above assumes that in the sheet named R in the workbook, the independent variable (team totals) are in column A and the dependent variable (ranks) are in column B.
The Forecast – The Third Place Value
The forecast function short circuits the work you have to do (instead of a formula that is SLOPE*10 + INTERCEPT) by forecasting (based on linear regression) what the desired independent variable value would be given a dependent variable value.
=FORECAST(10, R!A:A, R!B:B)
The calculation above assumes that in the sheet named R in the workbook, the independent variable (team totals) are in column A and the dependent variable (ranks) are in column B. The 10 as the first argument is the rank we are looking for the total value of based on our available data.
An Example Calculation
Category | Point Per Rank | Last Place | Third Place |
---|---|---|---|
R | 18 | 736 | 915 |
HR | 8 | 216 | 294 |
RBI | 19 | 690 | 882 |
SB | 6 | 50 | 107 |
AVG | 0.002 | 0.258 | 0.276 |
W | 4 | 57 | 99 |
SV | 9 | 11 | 99 |
K | 59 | 1020 | 1612 |
ERA | -0.093 | 4.631 | 3.697 |
WHIP | -0.017 | 1.343 | 1.175 |
For the 2020 fantasy baseball season, here are my targets for the Pitcher List staff leagues.
These targets are based entirely off of 2019 data across all 6 Pitcher List staff leagues. As stated earlier, the more history you have, the better your targets will be. 2019 will be heavily skewed by the ball which led to a startling increase in home runs. These 2020 targets, only including 2019 data, will reflect that environment, without other years to temper the expectations.
Putting It All Together
Once you’ve chosen a projection system (or a mix of your choice), you’ll want to track your draft based on the projected values of the players you’ve already chosen and your benchmarks. In years past, I have a spreadsheet with a line for each roster position and a totals row. Each time I select a player, I add their projection to the appropriate line and watch as the totals row updates. Below the total row, I typically add another row for my benchmarks, so I can see how I compare to each value.
In the last year or so, I have added a third row to the bottom that subtracts the running total from the benchmark and divides that by the number of empty roster spots (separated by batting and pitching) to calculate the average value needed for each unpicked player to meet my benchmark. This works fairly well for counting statistics, like Runs and Strike Outs.
Featured Image by Justin Paradis (@FreshMeatComm on Twitter)
Very Nice Work. Wish you would include OBP as well as AVG.
Take these numbers with a hefty grain of salt. Based on league results from across fantasy baseball that were supplied to me by other writers during the 2019 season, I was able to generate these values for OBP –
Last Place – 0.749
Per Rank – 0.0045
Third Place – 0.7941
Great article. Link to your 2020 pitcher targets doesn’t work. But now I will be even more lost in my spreadsheets. Thanks for the class. :)
Here is the full URL for the Google Sheet. https://docs.google.com/spreadsheets/d/1vlN29LgbYdU6-3nP94fSJY7bRvXRtw9ti6t9a3EpAOY/edit?usp=sharing
This is great! How do you reverse the intercept to get Last Place correct for categories where lower is better like ERA, WHIP, etc.
And also, do you change your target based on league size? So targeting 3rd for a 12 teamer but what about 14 teams?
I tend to target third for any league size, though to be perfectly honest, I haven’t actually done the math. I’ll try and remember this question when I revisit this subject to actually come up with an algorithm to choose the proper benchmark with larger league sizes.
It all starts with the calculation of RANK. The last parameter of the RANK function is whether or not the rank should be ascending or descending. Since we are looking for the lower values to have the higher rank, the rank needs to be descending, which is a 0 for that parameter. Since the slope of the line that would describe the graph is now negative thanks to the descending relationship of our value to its rank, the intercept will be appropriately placed to describe the line.
This is a fantastic article. Really great advice that’s well explained and applicable. Thanks! Can you do something similar, or apply these formulas/concepts using a pivot table?